Meta Learning Text-to-Speech Synthesis in over 7000 Languages

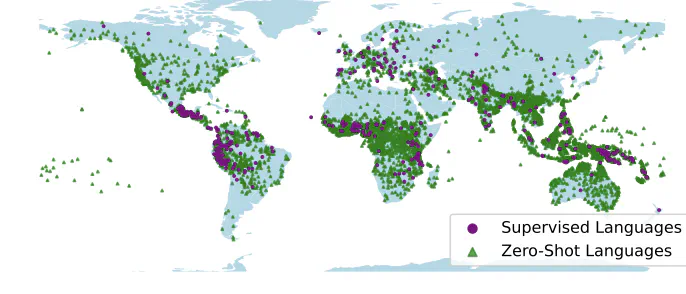
This research presents a novel meta-learning approach for text-to-speech synthesis that works across more than 7000 languages. By leveraging knowledge transfer between languages and a few-shot learning framework, our system can produce natural-sounding speech even for languages with extremely limited data resources.
The model demonstrates remarkable flexibility, adapting to diverse phonological systems, prosodic patterns, and writing systems. This advancement is particularly valuable for endangered and under-resourced languages, where commercial speech technology development is often not economically viable.
Our results show that with just a few minutes of speech data, the system can generate intelligible speech in previously unseen languages, opening new possibilities for language documentation, revitalization efforts, and accessible speech technology for all language communities regardless of size or economic status.