Enhancing Standard and Dialectal Frisian ASR: Multilingual Fine-tuning and Language Identification for Improved Low-resource Performance
Mar 7, 2025·,,,
,·
1 min read
Reihaneh Amooie
Wietse De Vries
Yun Hao
Jelske Dijkstra

Matt Coler
Martijn Wieling
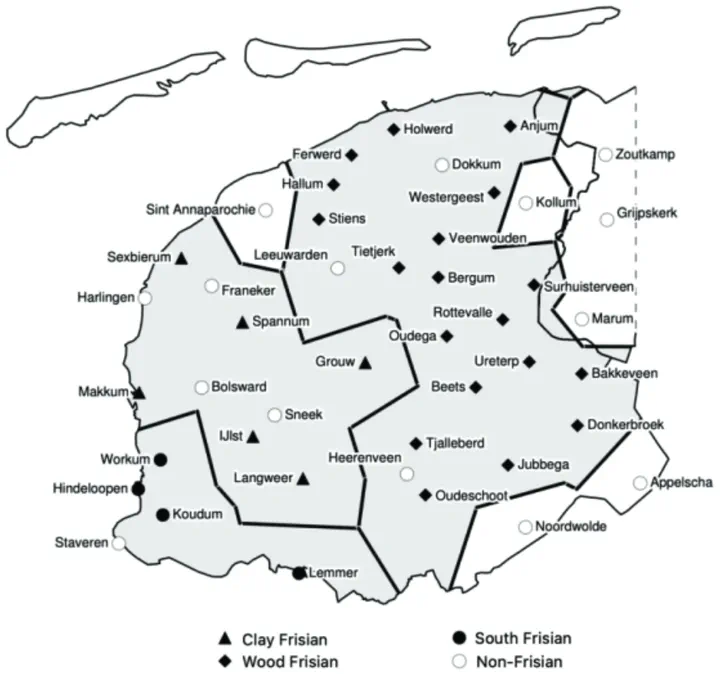
Abstract
Automatic Speech Recognition (ASR) performance for low-resource languages is still far behind that of higher-resource languages such as English, due to a lack of sufficient labeled data. State-of-the-art methods deploy self-supervised transfer learning where a model pre-trained on large amounts of data is fine-tuned using little labeled data in a target low-resource language. In this paper, we present and examine a method for fine-tuning an SSL-based model in order to improve the performance for Frisian and its regional dialects (Clay Frisian, Wood Frisian, and South Frisian). We show that Frisian ASR performance can be improved by using multilingual (Frisian, Dutch, English and German) fine-tuning data and an auxiliary language identification task. In addition, our findings show that performance on dialectal speech suffers substantially, and, importantly, that this effect is moderated by the elicitation approach used to collect the dialectal data. Our findings also particularly suggest that relying solely on standard language data for ASR evaluation may underestimate real-world performance, particularly in languages with substantial dialectal variation.
Type
Publication
IEEE International Conference on Acoustics, Speech and Signal Processing (ICASSP)
This research presents a method for enhancing ASR performance for Frisian and its regional dialects using multilingual fine-tuning and language identification. Key findings include:
- Adding Dutch and German data to the fine-tuning process improved Frisian ASR performance by approximately 7.5% relative error reduction
- Language identification tokens provided additional improvement, particularly for dialectal speech
- Performance was better on standard speech than dialectal speech
- Using Dutch texts for elicitation (which require translation) allowed for more natural regional variation than using standard Frisian texts
This work has implications for developing ASR systems for minority languages, as performance estimations based on standard language test sets may underestimate real-world performance when there is significant dialect variation.